What is WRAP and how can it help train AI more efficiently?
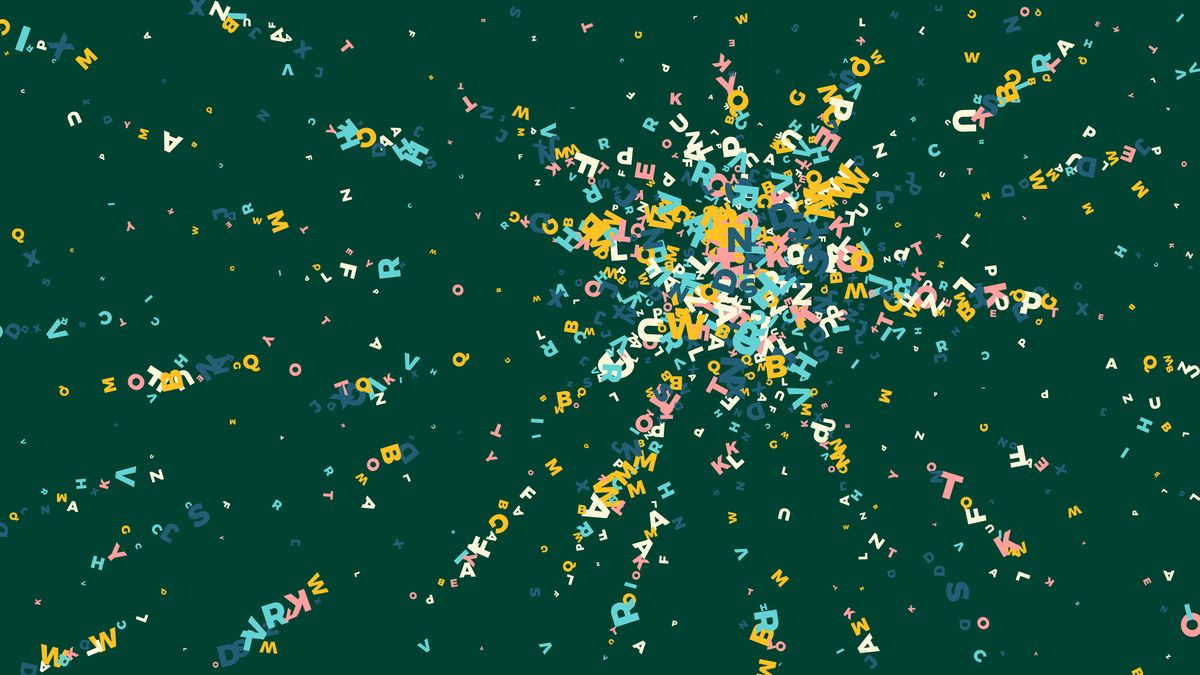
Generative AI is booming, though developers are quickly running into obstacles, from the high energy demands of AI compute to the complex infrastructure required to train systems.
For the latter, data is of the utmost importance. Stockpiles of clear, quality data are vital for those companies looking to train and build their own AI models. Getting data pools in order is a key part of the early development process.
One novel theory for making this process easier is web rephrase augmented pre-training (WRAP), a technique put forward by researchers at Apple and Carnegie Mellon University in a paper published earlier this year.
In it, the researchers noted that many large language models (LLMs) are trained on data scraped from the web that is often “unstructured, noisy, and poorly phrased,” making it harder to use for training.
While synthetic data can be used to get around this problem, it can fall victim to bias. While the alternative practice of data curation to remove lower-quality data can be effective, the researchers put forward their own solution.
In it, the researchers noted that many large language models (LLMs) are trained on data scraped from the web that is often “unstructured, noisy, and poorly phrased,” making it harder to use for training.
While synthetic data can be used to get around this problem, it can fall victim to bias. While the alternative practice of data curation to remove lower-quality data can be effective, the researchers put forward their own solution.
Rather than creating synthetic data, WRAP uses an “off-the-shelf instruction-tuned model prompted to paraphrase documents on the web in specific styles such as ‘like Wikipedia’ or in ‘question-answer format’ to jointly pre-train LLMs on real and synthetic rephrases.”
According to the report, WRAP sped up pretraining by about three times when used on a “naturally noisy” dataset.
How does WRAP work?
In the paper, researchers use the rephrasing process on ‘The Pile,’ a collection of datasets commonly used in AI according to senior research and development manager of data science at Synopsys, Dr Andrew Bolster.
“Some datasets are used as benchmarks to compare architectures and scales. One such collection of datasets is known as ‘The Pile,’” Bolster tells ITPro.
This is an 825GB collection of web scraped data, Bolster goes on, featuring content from a range of sites including PubMed, Github, Stack Exchange, HackerNews, and YouTube subtitles.
The researchers augment ‘The Pile’ by rephrasing large portions of it before combining these rephrased portions with the original dataset to train an LLM which answers questions, Bolster says. This LLM is then evalued for its zero-shot accuracy – meaning its capacity to answer questions not rooted in its training data.
“This is a form of ‘synthetic data augmentation’, where in any modeling system, there may not be sufficient ‘real’ input/training data to accurately converge a model’s behavior,” Bolster says.
“Data Scientists may simply ‘repeat’ the training data over and over again, hoping for the best, but over the past decade, this has largely been replaced with synthetic data generation involving the training of intermediate models to generate more data that ‘looks like’ the provided training set,” he adds.
The LLM trained on the rephrased data within the paper reportedly “outperforms other techniques” that fulfill the same need. There is a “small but clear improvement,” Bolster says.
WRAP appears to beat other natural language augmentation techniques such as synonym replacement and random word deletion, though Bolster is careful to point out that there are some evident downsides.
The pros and the cons of WRAP
For any businesses or developers looking to WRAP as a method of driving efficiency during AI model training, there are some key advantages and disadvantages to consider, particularly regarding proprietary data.
WRAP could work best for in-house AI training, says Stefan Leichenauer, vice president of engineering at SandboxAQ, but there may be “limited effectiveness out-of-the-box” when the method is applied to proprietary data.
“In-house training data may not be as naturally messy as what we find on the public internet,” Leichenauer tells ITPro. To fix this, he suggests businesses should convert their data into “something that is more in line with the end application we are interested in.”
“So, for example, if you are interested in training a customer service chatbot, then you should try transforming your internal documentation into a question-answer format before training the AI,” he says.
Messy data has always been a problem, Leichenauer adds, noting that WRAP is one of many tools developers can use to perform “initial data transformations” for more effective training.
On the other hand, Bolster notes that WRAP could cut the up-front costs of creating enterprise-grade LLMs, which is generally eaten up in the “establishment of curated / domain specific data”. In this sense, WRAP could have its advantages.
“This rephrase capability might be a valid method for making the most of limited data to train against,” Bolster says.
Having said that, WRAP has a “significant upfront cost” of its own – the generation of synthetic data. At present, there arealso sensitivities to rephrasing “styles” and model selection which could cause issues.
It’s clear that as WRAP matures, it could have a big impact on the way that companies pursue their own LLMs and refine their data to ensure the best ROI on their AI investments. Whether it will become as pivotal a development as the likes of retrieval-augmented generation (RAG) has yet to be seen, but businesses will be keenly investigating its benefits and drawbacks.